The Rise and Potential of Large Language Model-Based Agents: A Survey(Xi et. al.)
This survey paper explores the growing field of Large Language Model (LLM)-based agents, their potential, challenges, and future directions. LLM-based agents, such as GPT and BERT derivatives, have revolutionized AI by serving as general-purpose agents capable of reasoning, planning, and interacting across diverse domains. The paper covers their foundational advancements, applications, and societal impact.
RESEARCH
Shubhradeep
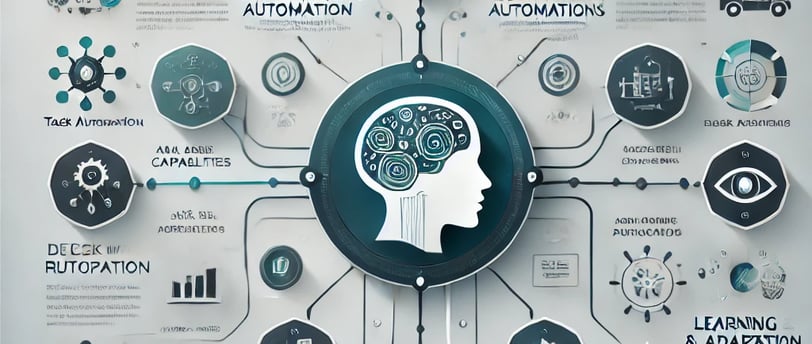
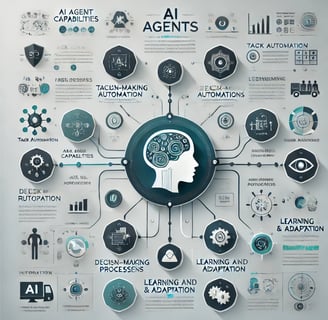
This survey paper explores the growing field of Large Language Model (LLM)-based agents, their potential, challenges, and future directions. LLM-based agents, such as GPT and BERT derivatives, have revolutionized AI by serving as general-purpose agents capable of reasoning, planning, and interacting across diverse domains. The paper covers their foundational advancements, applications, and societal impact.
Foundations of LLM-Based Agents
Core Innovations: The rise of LLMs stems from advancements in Transformer architectures, self-supervised learning, and massive-scale training on diverse datasets.
Capabilities: These agents can generate human-like text, summarize information, answer questions, translate languages, and even perform basic reasoning.
Fine-Tuning and Adaptation: Techniques like transfer learning, prompt engineering, and low-rank adaptation (e.g., LoRA) have extended the utility of LLMs.
Applications Across Domains
Education: Personalized tutoring, question answering, and automated grading.
Healthcare: Symptom analysis, medical record summarization, and health chatbots.
Business: Customer service automation, content generation, and market analysis.
Creative Fields: Writing, art, and music generation powered by multimodal LLMs.
Challenges
Scalability: Training and deploying LLMs require significant computational and energy resources.
Hallucination: LLMs occasionally generate inaccurate or fabricated information.
Ethics and Bias: Concerns include reinforcing societal biases, generating harmful content, and intellectual property infringement.
Real-World Integration: Adapting LLMs for specific applications often requires fine-tuning and task-specific customization.
Societal Implications
Positive Impact: Enhancing productivity, democratizing AI capabilities, and improving accessibility in low-resource settings.
Risks: Job displacement, misinformation propagation, and misuse by malicious actors.
Future Directions
Alignment: Developing better alignment techniques to ensure outputs are accurate and ethical.
Efficient Training: Innovations like LoRA and parameter-efficient fine-tuning to reduce costs.
Interdisciplinary Collaboration: Integrating LLMs with robotics, vision, and other modalities to create multi-functional agents.
Open Research: Encouraging transparency, reproducibility, and ethical AI practices.
Conclusion: LLM-based agents have immense transformative potential across industries, but realizing their full benefits requires addressing technical, ethical, and societal challenges. The survey underscores the importance of collaborative innovation to harness the promise of LLMs responsibly.
AI430 - AI Agent HUB
Powering your Gen AI Journey
Email Us
Contact us
kalpita@ai430.com
© 2025. All rights reserved.
Call back